The explosion of Web of Issues (IoT) gadgets has remodeled our world in numerous methods, from sensible factories to related healthcare programs. Based on latest projections by IoT Analytics, the variety of related IoT gadgets is anticipated to succeed in 40 billion by 2030 [1]. This exponential development has created an expansive and infrequently invisible assault floor that conventional safety measures battle to guard.
The problem is evident: how will we safe networks populated by hundreds of various IoT gadgets, every doubtlessly serving as an entry level for risk actors? The reply more and more lies in anomaly detection—the potential to establish uncommon patterns that deviate from anticipated conduct inside IoT ecosystems.
The Distinctive Safety Challenges of IoT Networks
IoT environments current distinct cybersecurity challenges that make them notably weak to assaults:
Useful resource Constraints
Many IoT gadgets function with minimal computational assets, making conventional endpoint safety options impractical. Analysis reveals that shopper IoT gadgets lack fundamental safety capabilities resulting from {hardware} limitations.
Heterogeneous Ecosystems
Not like conventional IT environments with standardized programs, IoT networks sometimes incorporate gadgets from quite a few producers with various protocols, working programs, and safety requirements. This heterogeneity complicates uniform safety implementation.
Operational Criticality
In industrial settings, healthcare, or important infrastructure, IoT gadgets usually management bodily operations the place safety failures may have extreme real-world penalties past knowledge loss.
Navigate the complexities of NDR options and discover the perfect match in your safety wants!
What’s Contained in the Purchaser’s Information?
-
Key options to search for -
The best way to consider the software -
Insights on evolving threats
IoT Anomaly Detection: The Basis of Trendy Safety
Anomaly detection has emerged as a cornerstone know-how for safeguarding IoT ecosystems. By establishing behavioral baselines for networks, gadgets, and visitors patterns, organizations can establish deviations which will point out compromise.
How IoT Anomaly Detection Works
At its core, IoT anomaly detection entails three elementary phases:
- Studying Part: The system analyzes community visitors, machine conduct, and communication patterns to determine a baseline of “regular” operations.
- Detection Part: Steady monitoring compares present exercise towards established baselines to establish deviations.
- Response Part: When anomalies are detected, the system triggers alerts or automated responses based mostly on predefined guidelines and danger assessments.
Probably the most refined IoT safety programs don’t depend on static guidelines alone however make use of dynamic behavioral modeling to adapt to evolving community situations whereas nonetheless figuring out official anomalies.
Key Methods in IoT Anomaly Detection
A number of approaches have confirmed efficient in figuring out suspicious conduct in IoT environments:
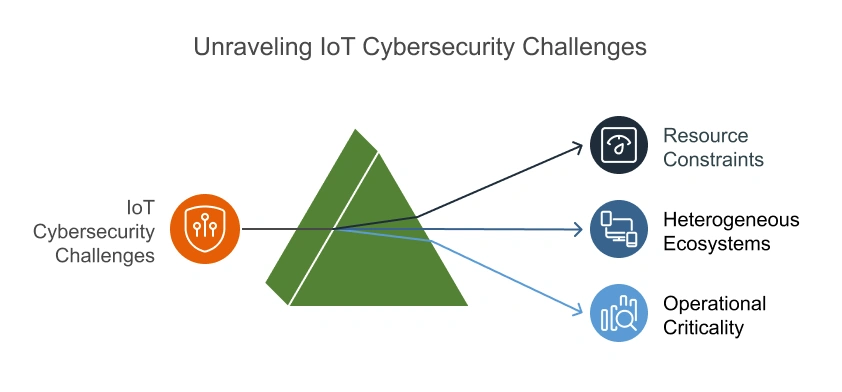
Statistical Strategies
Statistical approaches analyze historic knowledge to determine regular behavioral patterns. Deviations past statistical thresholds set off alerts. These strategies work properly for steady IoT deployments with predictable operational patterns.
The problem with purely statistical strategies is establishing applicable thresholds that reduce false positives whereas catching real threats. A research on anomaly detection in cybersecurity utilizing AI strategies discusses the challenges of excessive false optimistic charges related to conventional statistical strategies[2].
Machine Studying
Machine studying has revolutionized anomaly detection in IoT gadgets by enabling programs to establish complicated patterns that might be not possible to program manually. Key ML approaches embody:
- Supervised Studying: Fashions are skilled on labeled datasets containing examples of regular and anomalous conduct.
- Unsupervised Studying: Methods establish clusters and patterns in unlabeled knowledge to detect outliers with out prior examples of assaults.
- Deep Studying: Neural networks analyze complicated temporal patterns in IoT time sequence knowledge to establish delicate anomalies that may escape detection by less complicated fashions.
Behavioral Evaluation
Behavioral evaluation focuses on understanding the anticipated communication patterns and actions of gadgets. By modeling the standard conduct of every machine kind, safety programs can flag surprising actions, resembling:
- A wise thermostat out of the blue trying to entry monetary programs
- An industrial sensor transmitting knowledge at uncommon instances
- Linked gadgets speaking with identified malicious IP addresses
- Sudden firmware updates or configuration modifications
Hybrid Approaches
The best anomaly detection programs for IoT networks mix a number of detection strategies. Analysis reveals organizations implementing hybrid approaches expertise fewer profitable breaches in comparison with these counting on a single detection methodology[2].
Anomaly Detection Fashions for IoT Time Sequence Knowledge
IoT gadgets generate huge quantities of time-series knowledge—sequential knowledge factors collected at common intervals. This knowledge presents each challenges and alternatives for anomaly detection.
Time Sequence-Particular Fashions
A number of specialised fashions have demonstrated specific efficacy with IoT time sequence knowledge:
- LSTM (Lengthy Quick-Time period Reminiscence) Networks: These neural networks excel at studying patterns in sequential knowledge and may detect anomalies in time sequence by predicting anticipated values and evaluating them to precise readings.
- Autoencoder Fashions: By compressing and reconstructing enter knowledge, autoencoders can establish anomalies that don’t reconstruct correctly, indicating deviation from realized patterns.
- GAN (Generative Adversarial Community) Primarily based Fashions: These fashions be taught to generate “regular” knowledge patterns and may establish actual knowledge that differs considerably from the generated examples.
IoT Anomaly Detection Datasets
Growing efficient anomaly detection requires intensive testing with consultant datasets. A number of public IoT anomaly detection datasets have grow to be commonplace benchmarks for creating and evaluating fashions:
Main Public Datasets
- N-BaIoT: Accommodates knowledge from actual IoT gadgets contaminated with Mirai and BASHLITE malware, permitting researchers to check detection of precise malware conduct.
- TON_IoT: A complete dataset collected on the Cyber Vary Lab of UNSW Canberra, containing telemetry from IoT gadgets, Home windows community visitors, and Linux datasets with numerous assault eventualities.
- Edge-IIoTset: Centered on industrial IoT environments, this dataset incorporates each regular operations and numerous assault eventualities particularly concentrating on edge computing in industrial settings.
- WUSTL-EHMS: Accommodates knowledge from a real-world sensible dwelling atmosphere with official person actions and simulated assaults.
Implementation Challenges and Options
Regardless of its effectiveness, implementing IoT anomaly detection presents a number of challenges:
False Positives
Overly delicate detection programs can generate alert fatigue, inflicting safety groups to grow to be desensitized to warnings.
Resolution: Superior correlation strategies that group associated alerts and supply context. Trendy NDR options like Fidelis Community® routinely group associated alerts to avoid wasting important time whereas offering malware evaluation and enhancing risk looking capabilities. Their resolution offers customers aggregated alerts, context, and proof for sooner risk investigation, deeper evaluation, and lowered alert fatigue.
Encrypted Visitors
The growing use of encryption in IoT communications can blind conventional monitoring options.
Resolution: Superior programs can analyze encrypted visitors patterns with out decryption. Profiling TLS encrypted visitors capabilities that differentiate between human shopping versus machine visitors and use evolving knowledge science fashions to detect hidden threats even in encrypted communications.
Scale and Efficiency
Processing huge quantities of IoT telemetry requires vital computational assets.
Resolution: Distributed processing architectures and edge computing. Based on the documentation, Fidelis Community® makes use of quick knowledge processing capabilities with minimal rack area necessities (20GB 1U Sensor) to deal with enterprise-scale deployments.
Safe your IoT ecosystem like by no means earlier than!
What’s Contained in the Datasheet?
-
How Fidelis Community® makes use of ML for anomaly detection -
Actual-time risk detection -
Key integrations
Actual-World Implementation: A Framework
Organizations implementing IoT anomaly detection ought to observe a structured strategy:
- Asset Discovery and Classification: Preserve a complete stock of all IoT gadgets on the community.
- Baseline Institution: Monitor regular operations for every machine kind to know typical conduct patterns.
- Mannequin Choice and Deployment: Select applicable detection fashions based mostly in your atmosphere and deploy monitoring throughout the community.
- Alert Tuning: Refine detection thresholds to reduce false positives whereas sustaining sensitivity to real threats.
- Integration: Join anomaly detection programs with broader safety ecosystems for coordinated response.
Community Detection and Response: The Broader Context
IoT anomaly detection features most successfully as a part of a complete Community Detection and Response (NDR) technique. NDR options present the broader context and response capabilities wanted to transform anomaly detection into actionable safety.
NDR options have advanced to establish and thwart network-related threats that you simply may not be capable of block utilizing older programs which often depend upon identified assault patterns and signatures. They detect threats, dangerous conduct and malicious actions on enterprise networks utilizing non-signature-based strategies like machine studying and synthetic intelligence.
The Fidelis Strategy to IoT Safety
Fidelis Community®, a part of the Fidelis Elevate XDR platform, gives a number of capabilities notably related to securing IoT environments:
- Deep Session Inspection: The patented resolution that appears deep into nested information gives wealthy content material with context for deeper evaluation. That is essential for IoT environments the place malicious content material may be hidden inside seemingly benign communications.
- Behavioral Evaluation: Fidelis Community® employs community conduct evaluation to detect anomalous patterns that may point out compromise, notably necessary for IoT gadgets that sometimes observe common communication patterns.
- Machine Studying: The answer makes use of machine-learning based mostly anomaly detection to establish uncommon conduct that may escape rule-based detection programs.
- MITRE ATT&CK Framework Mapping: Threats are mapped towards the MITRE ATT&CK framework, offering safety groups with a standardized understanding of assault strategies being employed.
- A number of Deployment Choices: Fidelis Community® gives versatile deployment via on-premises {hardware}; digital machine (VMware) assist; Cloud deployment (buyer or Fidelis Safety managed), accommodating the various infrastructure necessities of IoT implementations.
Conclusion: The Way forward for IoT Safety
As organizations proceed to broaden their IoT deployments, anomaly detection will stay a important safety element. Trying forward, a number of tendencies will form the evolution of this know-how:
- AI-Pushed Automation: More and more refined AI fashions will enhance detection accuracy whereas decreasing human intervention necessities.
- Edge-Primarily based Detection: Extra detection capabilities will transfer to the community edge to cut back latency and bandwidth necessities.
- Zero Belief Integration: Anomaly detection will grow to be a core element of Zero Belief architectures, offering steady validation of machine conduct.
- Regulatory Compliance: Rising IoT safety laws will probably mandate anomaly detection capabilities for important programs.
Organizations that implement sturdy anomaly detection as a part of their broader safety technique will probably be greatest positioned to safe their rising IoT ecosystems towards more and more refined threats.
With the appropriate NDR resolution, your group can successfully forestall cyber-attacks and preserve adversaries away out of your networks—a aim that turns into ever extra important as our world turns into more and more related.
Steadily Ask Questions
What makes IoT anomaly detection totally different from conventional community safety?
IoT safety isn’t simply conventional community safety with a brand new title slapped on it. The variations run deep.
IoT environments are a large number of various gadgets, every talking their very own language and following their very own guidelines. You’ve acquired every little thing from industrial sensors to sensible lightbulbs making an attempt to coexist.
Most of those devices work with minimal computing energy – they’re constructed to do one job cheaply, not run safety software program. The upside? They often observe predictable patterns, making uncommon conduct simpler to identify if you realize what to search for.
And let’s speak scale. Whenever you’re monitoring hundreds or hundreds of thousands of gadgets, you want programs that may deal with that firehose of information with out choking.
How lengthy does it take to determine a dependable behavioral baseline for IoT gadgets?
There’s no one-size-fits-all reply right here. It actually is determined by what you’re monitoring.
For predictable environments like factories or utilities, you would possibly get stable baselines in simply 2-4 weeks. The machines do the identical issues day in, time out.
However retail shops, workplace buildings, or something with seasonal patterns? You’re 1-3 months minimal. You have to seize these weekly conferences, month-to-month stock cycles, or quarterly peak durations.
Throughout this studying part, anticipate to roll up your sleeves and fine-tune these sensitivity settings. Too delicate and also you’ll drown in false alarms; too lax and also you’ll miss the true threats.
The publish Anomaly Detection in IoT Networks: Securing the Unseen Perimeter appeared first on Fidelis Safety.