We see knowledge visualizations in every single place: studies, displays, social media, and even the information. But have you ever ever seen a chart that simply didn’t make sense? Regardless of how frequent they’re, selecting the fitting chart isn’t at all times intuitive. The secret’s to begin by clearly figuring out the query you wish to reply.
On this tutorial, we’ll train you ways to decide on the very best visualization to reply your knowledge questions. By the tip, you may have the boldness and readability wanted to pick efficient charts that talk your insights clearly and persuasively.
Why Selecting the Proper Chart Begins with Your Query
We’ll be utilizing transaction knowledge from a fictional retail enterprise, “Superstore,” which sells furnishings and electronics. Whereas the info isn’t actual, it displays frequent enterprise eventualities, making it a helpful studying device. The method of choosing the proper visualization stays the identical whether or not we’re working with actual firm knowledge or a follow dataset.
However earlier than we are able to create an efficient visualization, we have to outline what we’re making an attempt to know. In any other case, even a well-designed chart can result in deceptive conclusions.
Think about now we have knowledge on low cost percentages and earnings. With no clear query, we would choose a chart at random—say, a pie chart—and find yourself with a complicated visible. Pie charts present components of a complete, not relationships between numeric variables.
As a substitute, if we ask “How do low cost percentages have an effect on revenue?” we see that we’re searching for a relationship between two numbers. A scatter plot turns into the plain alternative, revealing whether or not greater reductions result in decrease earnings.
Beginning with a transparent query ensures that your chart alternative aligns together with your purpose, making it simpler to your viewers to know the insights you wish to share.
Matching Your Query to the Proper Chart
Now, we’ll break down seven frequent chart sorts one after the other, specializing in the questions they reply greatest.
1. Bar Charts: Evaluating Classes
When our knowledge query is about evaluating discrete classes (distinct teams or sorts, resembling completely different merchandise, areas, or departments), a bar chart is usually the only option. As a result of every bar’s size is straight comparable, bar charts make it straightforward to see which classes are bigger or smaller at a look, highlighting variations in magnitude.
Reply questions like:
❔ How do completely different [categories] examine by way of [measurement]?
Superstore Instance:
We wish to present which merchandise generated the best gross sales at Superstore. A bar chart clearly compares whole gross sales throughout completely different merchandise, instantly highlighting the standout performers, just like the Nokia Good Telephone or Novimex government leather-based armchair.
✅
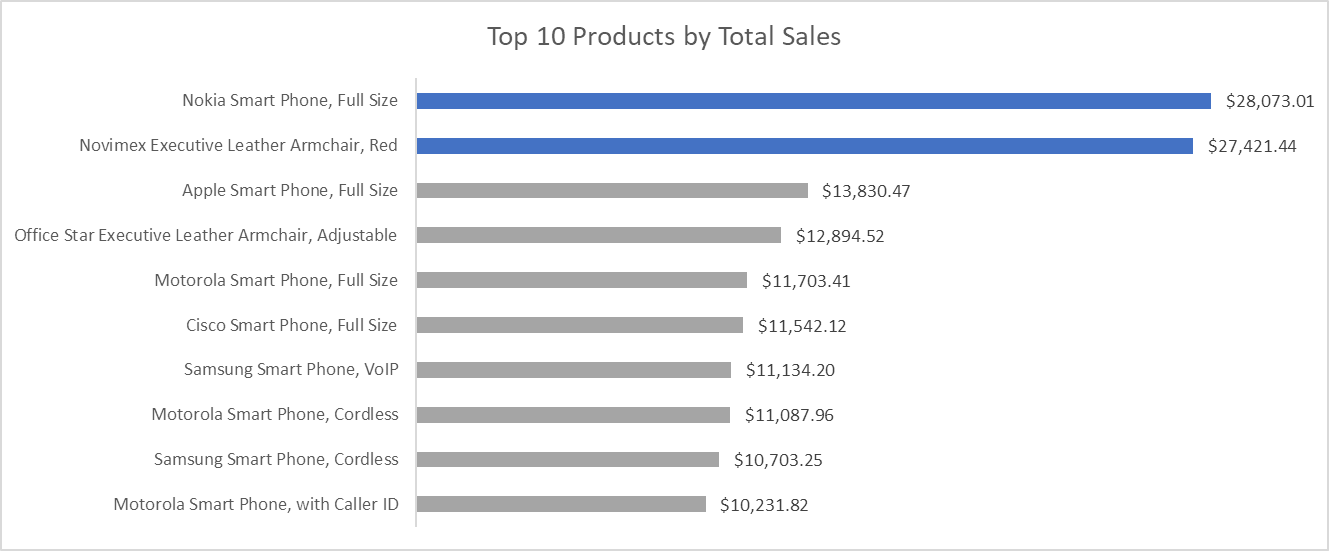
Nonetheless, bar charts work greatest when evaluating an affordable variety of classes, ideally not more than 15. Past that, the chart turns into cluttered, making it onerous to learn labels and examine values.
Within the instance beneath, the bars turn into troublesome to interpret as a result of too many classes are squeezed into the chart. The Nokia Good Telephone and the Novimex Armchair are technically the very best performers, however their labels are misplaced within the visible noise, making it tougher to attract significant conclusions.
❌
If we’re evaluating discrete classes, a bar chart gives the clearest view. But when we’re exploring relationships between numeric variables or adjustments over time, contemplate a scatter plot or line chart as an alternative.
2. Line Charts: Exhibiting Modifications Over Time
If a knowledge query includes understanding how knowledge adjustments over a steady interval, particularly time, a line chart is a good visualization to make use of. Line charts clearly illustrate tendencies, patterns, or fluctuations, making it straightforward for viewers to trace adjustments over time.
Reply questions like:
❔ How has [measurement] modified over [time period]?
Superstore Instance:
We’re analyzing month-to-month gross sales knowledge over a number of years and ask ourselves, “What are the tendencies in gross sales over time?” A line chart instantly reveals tendencies, resembling intervals of constant development or seasonal peaks and valleys. Our viewers can rapidly perceive how gross sales efficiency has advanced, permitting us to debate future methods primarily based on clearly seen patterns.
If we have to examine a number of collection (for instance, gross sales in several areas over time), we are able to plot a number of strains on the identical chart. Simply remember to label them clearly or use a legend to keep away from confusion.
Nonetheless, don’t use line charts when evaluating unrelated classes. Line charts indicate continuity and sequential development, which may be deceptive when classes aren’t naturally related or ordered.
3. Scatter Plots: Exploring Relationships Between Numeric Variables
When now we have a query about how two numeric variables relate to one another, we should always instantly consider scatter plots.
How they work:
A scatter plot locations every knowledge level on a two-dimensional grid, with the variable we suspect influences the opposite (the unbiased or predictor variable) on the x-axis, and the variable we wish to measure or predict (the dependent or end result variable) on the y-axis. This setup permits us to see patterns which may not be apparent in a desk of numbers. Clusters of factors counsel frequent combos or correlation course and energy, whereas outliers draw consideration to uncommon instances which may want additional investigation.
If we suspect a correlation or wish to measure it, we are able to contemplate including a pattern line (or regression line) to spotlight the connection extra clearly. We are able to additionally add a third variable through the use of coloration, dimension, or form to distinguish knowledge factors—revealing even deeper insights into the info.
Reply questions like:
❔ How does [numeric variable A] relate to [numeric variable B]?
Superstore Instance:
If our query is, “How does the low cost proportion have an effect on profitability?” a scatter plot clearly illustrates this relationship. Every dot represents a person sale, revealing patterns resembling greater reductions usually leading to decrease earnings.
On this instance, the scatter plot reveals one thing fascinating. We see that reductions of 15% or decrease keep first rate revenue margins, whereas greater reductions typically dip into destructive territory.
This perception can be practically unattainable to identify in a knowledge desk or a easy abstract statistic. The scatter plot makes it instantly seen and actionable.
4. Histograms: Understanding the Distribution of Numeric Information
Histograms are an awesome alternative after we’re asking concerning the distribution or frequency of numerical knowledge.
How they work:
Histograms group knowledge factors into ranges, or “bins,” illustrating what number of knowledge factors fall into every numeric interval. By adjusting the variety of bins, we are able to spotlight broader patterns or zoom in on finer particulars. However watch out: too many bins could make the chart look noisy, whereas too few can clean over vital nuances. A great place to begin is round 10 to twenty bins, relying on how a lot knowledge you’ve got. If the histogram seems too jagged or too smoothed out, attempt growing or reducing the variety of bins till the sample turns into clear.
Usually, the y-axis represents the frequency or depend of information factors in every bin, and the x-axis represents the numeric vary.
We are able to additionally achieve useful perception into the form of our knowledge. A single peak may point out a standard distribution, whereas a number of peaks or a powerful skew might counsel deeper tendencies or knowledge high quality points.
Reply questions like:
❔ What’s the distribution of [numeric variable]?
Superstore Instance:
We’re taking a look at knowledge on order portions and ask ourselves, “What is the typical dimension of buyer orders?” A histogram instantly reveals that the majority orders include between one and 5 gadgets. This perception helps us with stock planning: if most orders are small, stocking giant bundles may not be the very best technique.
Histograms vs. Bar Charts
At first, histograms can appear to be bar charts, however every chart serves a distinct goal. Histograms show numeric knowledge grouped into steady bins, which is beneficial after we wish to see the distribution of values. In distinction, bar charts are used for categorical knowledge, the place every bar represents a separate class (resembling product sorts or areas). Mixing them up can confuse your viewers about whether or not the info is steady or categorical.
5. Stacked Bar Charts: Exhibiting Half-to-Complete Relationships
Stacked bar charts work properly when you might want to present how completely different subcategories contribute to a complete. Every bar clearly represents the overall worth, with segments exhibiting the contribution of every subcategory.
Reply questions like:
❔ How do subcategories contribute to every class’s whole?
Superstore Instance:
We would use a stacked bar chart to reply, “How do gross sales differ by area and buyer section?” This visible clearly demonstrates how areas differ and the way every buyer section contributes to regional gross sales totals. It supplies an instantaneous sense of proportion, highlighting the segments driving general gross sales efficiency. At a look we are able to see that the Central
area drives essentially the most gross sales and that the Client
section accounts for the most important proportion of gross sales for each area.
6. Pie Charts: Clearly Exhibiting Proportions
Pie charts work properly for questions on proportions as a result of they clearly present how particular person classes contribute to a complete. They work greatest when all of your classes sum to a significant complete (usually 100%). If our knowledge doesn’t signify a whole set of components, a pie chart can mislead the viewers concerning the general composition.
Reply questions like:
❔ What quantity of the overall does every class signify?
Superstore Instance:
If we ask, “What quantity of whole gross sales comes from every product class?” a pie chart successfully illustrates that classes resembling telephones, chairs, and bookcases dominate general gross sales. The proportions are instantly clear and intuitive. We are able to see that telephones and bookcases make up practically half of all gross sales, whereas subcategories like tables and furnishings account for a a lot smaller proportion.
Pie charts are easy for exhibiting just a few giant classes, however they turn into onerous to interpret when you’ve got many slices or want shut comparisons. In these instances, bar charts or stacked bar charts typically work higher for readability.
7. Field Plots: Highlighting Distribution and Outliers
Field plots (also referred to as box-and-whisker plots) are wonderful for questions on evaluating how knowledge is distributed throughout classes. They show the median, interquartile vary (the “field”), and potential outliers (the “whiskers”). By rapidly exhibiting the unfold of values, field plots assist us determine extremes and gauge general consistency.
Reply questions like:
❔ How does the distribution of [numeric variable] examine throughout completely different [categories]?
Superstore Instance:
Our query is: “Do revenue margins differ considerably by area, and which area has the widest unfold?” As a result of this query focuses on evaluating the distribution of a numeric variable throughout teams, a field plot is a perfect alternative. After we take a look at the chart, we see that the North has revenue margins starting from round –$200 to $350, with a median close to $50. Central is extra tightly clustered round its median, and the South reveals a reasonable vary, with dips beneath zero. These variations assist us determine variation, spot potential outliers, and determine the place to research additional.
Field plots are highly effective for evaluating distributions throughout a number of teams. Nonetheless, in case your viewers isn’t accustomed to them, present a short rationalization or go for histograms when exhibiting a single numeric distribution.
Evaluate
Selecting the best visualization at all times begins by clearly defining the query you are making an attempt to reply. Totally different questions naturally result in completely different chart sorts, and clearly matching your visualization to your query is crucial for efficient communication.
With follow, choosing the fitting visualization will turn into second nature. You will really feel assured figuring out you are clearly speaking your knowledge insights, making your visuals straightforward for any viewers to know and act upon.
We see knowledge visualizations in every single place: studies, displays, social media, and even the information. But have you ever ever seen a chart that simply didn’t make sense? Regardless of how frequent they’re, selecting the fitting chart isn’t at all times intuitive. The secret’s to begin by clearly figuring out the query you wish to reply.
On this tutorial, we’ll train you ways to decide on the very best visualization to reply your knowledge questions. By the tip, you may have the boldness and readability wanted to pick efficient charts that talk your insights clearly and persuasively.
Why Selecting the Proper Chart Begins with Your Query
We’ll be utilizing transaction knowledge from a fictional retail enterprise, “Superstore,” which sells furnishings and electronics. Whereas the info isn’t actual, it displays frequent enterprise eventualities, making it a helpful studying device. The method of choosing the proper visualization stays the identical whether or not we’re working with actual firm knowledge or a follow dataset.
However earlier than we are able to create an efficient visualization, we have to outline what we’re making an attempt to know. In any other case, even a well-designed chart can result in deceptive conclusions.
Think about now we have knowledge on low cost percentages and earnings. With no clear query, we would choose a chart at random—say, a pie chart—and find yourself with a complicated visible. Pie charts present components of a complete, not relationships between numeric variables.
As a substitute, if we ask “How do low cost percentages have an effect on revenue?” we see that we’re searching for a relationship between two numbers. A scatter plot turns into the plain alternative, revealing whether or not greater reductions result in decrease earnings.
Beginning with a transparent query ensures that your chart alternative aligns together with your purpose, making it simpler to your viewers to know the insights you wish to share.
Matching Your Query to the Proper Chart
Now, we’ll break down seven frequent chart sorts one after the other, specializing in the questions they reply greatest.
1. Bar Charts: Evaluating Classes
When our knowledge query is about evaluating discrete classes (distinct teams or sorts, resembling completely different merchandise, areas, or departments), a bar chart is usually the only option. As a result of every bar’s size is straight comparable, bar charts make it straightforward to see which classes are bigger or smaller at a look, highlighting variations in magnitude.
Reply questions like:
❔ How do completely different [categories] examine by way of [measurement]?
Superstore Instance:
We wish to present which merchandise generated the best gross sales at Superstore. A bar chart clearly compares whole gross sales throughout completely different merchandise, instantly highlighting the standout performers, just like the Nokia Good Telephone or Novimex government leather-based armchair.
✅
Nonetheless, bar charts work greatest when evaluating an affordable variety of classes, ideally not more than 15. Past that, the chart turns into cluttered, making it onerous to learn labels and examine values.
Within the instance beneath, the bars turn into troublesome to interpret as a result of too many classes are squeezed into the chart. The Nokia Good Telephone and the Novimex Armchair are technically the very best performers, however their labels are misplaced within the visible noise, making it tougher to attract significant conclusions.
❌
If we’re evaluating discrete classes, a bar chart gives the clearest view. But when we’re exploring relationships between numeric variables or adjustments over time, contemplate a scatter plot or line chart as an alternative.
2. Line Charts: Exhibiting Modifications Over Time
If a knowledge query includes understanding how knowledge adjustments over a steady interval, particularly time, a line chart is a good visualization to make use of. Line charts clearly illustrate tendencies, patterns, or fluctuations, making it straightforward for viewers to trace adjustments over time.
Reply questions like:
❔ How has [measurement] modified over [time period]?
Superstore Instance:
We’re analyzing month-to-month gross sales knowledge over a number of years and ask ourselves, “What are the tendencies in gross sales over time?” A line chart instantly reveals tendencies, resembling intervals of constant development or seasonal peaks and valleys. Our viewers can rapidly perceive how gross sales efficiency has advanced, permitting us to debate future methods primarily based on clearly seen patterns.
If we have to examine a number of collection (for instance, gross sales in several areas over time), we are able to plot a number of strains on the identical chart. Simply remember to label them clearly or use a legend to keep away from confusion.
Nonetheless, don’t use line charts when evaluating unrelated classes. Line charts indicate continuity and sequential development, which may be deceptive when classes aren’t naturally related or ordered.
3. Scatter Plots: Exploring Relationships Between Numeric Variables
When now we have a query about how two numeric variables relate to one another, we should always instantly consider scatter plots.
How they work:
A scatter plot locations every knowledge level on a two-dimensional grid, with the variable we suspect influences the opposite (the unbiased or predictor variable) on the x-axis, and the variable we wish to measure or predict (the dependent or end result variable) on the y-axis. This setup permits us to see patterns which may not be apparent in a desk of numbers. Clusters of factors counsel frequent combos or correlation course and energy, whereas outliers draw consideration to uncommon instances which may want additional investigation.
If we suspect a correlation or wish to measure it, we are able to contemplate including a pattern line (or regression line) to spotlight the connection extra clearly. We are able to additionally add a third variable through the use of coloration, dimension, or form to distinguish knowledge factors—revealing even deeper insights into the info.
Reply questions like:
❔ How does [numeric variable A] relate to [numeric variable B]?
Superstore Instance:
If our query is, “How does the low cost proportion have an effect on profitability?” a scatter plot clearly illustrates this relationship. Every dot represents a person sale, revealing patterns resembling greater reductions usually leading to decrease earnings.
On this instance, the scatter plot reveals one thing fascinating. We see that reductions of 15% or decrease keep first rate revenue margins, whereas greater reductions typically dip into destructive territory.
This perception can be practically unattainable to identify in a knowledge desk or a easy abstract statistic. The scatter plot makes it instantly seen and actionable.
4. Histograms: Understanding the Distribution of Numeric Information
Histograms are an awesome alternative after we’re asking concerning the distribution or frequency of numerical knowledge.
How they work:
Histograms group knowledge factors into ranges, or “bins,” illustrating what number of knowledge factors fall into every numeric interval. By adjusting the variety of bins, we are able to spotlight broader patterns or zoom in on finer particulars. However watch out: too many bins could make the chart look noisy, whereas too few can clean over vital nuances. A great place to begin is round 10 to twenty bins, relying on how a lot knowledge you’ve got. If the histogram seems too jagged or too smoothed out, attempt growing or reducing the variety of bins till the sample turns into clear.
Usually, the y-axis represents the frequency or depend of information factors in every bin, and the x-axis represents the numeric vary.
We are able to additionally achieve useful perception into the form of our knowledge. A single peak may point out a standard distribution, whereas a number of peaks or a powerful skew might counsel deeper tendencies or knowledge high quality points.
Reply questions like:
❔ What’s the distribution of [numeric variable]?
Superstore Instance:
We’re taking a look at knowledge on order portions and ask ourselves, “What is the typical dimension of buyer orders?” A histogram instantly reveals that the majority orders include between one and 5 gadgets. This perception helps us with stock planning: if most orders are small, stocking giant bundles may not be the very best technique.
Histograms vs. Bar Charts
At first, histograms can appear to be bar charts, however every chart serves a distinct goal. Histograms show numeric knowledge grouped into steady bins, which is beneficial after we wish to see the distribution of values. In distinction, bar charts are used for categorical knowledge, the place every bar represents a separate class (resembling product sorts or areas). Mixing them up can confuse your viewers about whether or not the info is steady or categorical.
5. Stacked Bar Charts: Exhibiting Half-to-Complete Relationships
Stacked bar charts work properly when you might want to present how completely different subcategories contribute to a complete. Every bar clearly represents the overall worth, with segments exhibiting the contribution of every subcategory.
Reply questions like:
❔ How do subcategories contribute to every class’s whole?
Superstore Instance:
We would use a stacked bar chart to reply, “How do gross sales differ by area and buyer section?” This visible clearly demonstrates how areas differ and the way every buyer section contributes to regional gross sales totals. It supplies an instantaneous sense of proportion, highlighting the segments driving general gross sales efficiency. At a look we are able to see that the Central
area drives essentially the most gross sales and that the Client
section accounts for the most important proportion of gross sales for each area.
6. Pie Charts: Clearly Exhibiting Proportions
Pie charts work properly for questions on proportions as a result of they clearly present how particular person classes contribute to a complete. They work greatest when all of your classes sum to a significant complete (usually 100%). If our knowledge doesn’t signify a whole set of components, a pie chart can mislead the viewers concerning the general composition.
Reply questions like:
❔ What quantity of the overall does every class signify?
Superstore Instance:
If we ask, “What quantity of whole gross sales comes from every product class?” a pie chart successfully illustrates that classes resembling telephones, chairs, and bookcases dominate general gross sales. The proportions are instantly clear and intuitive. We are able to see that telephones and bookcases make up practically half of all gross sales, whereas subcategories like tables and furnishings account for a a lot smaller proportion.
Pie charts are easy for exhibiting just a few giant classes, however they turn into onerous to interpret when you’ve got many slices or want shut comparisons. In these instances, bar charts or stacked bar charts typically work higher for readability.
7. Field Plots: Highlighting Distribution and Outliers
Field plots (also referred to as box-and-whisker plots) are wonderful for questions on evaluating how knowledge is distributed throughout classes. They show the median, interquartile vary (the “field”), and potential outliers (the “whiskers”). By rapidly exhibiting the unfold of values, field plots assist us determine extremes and gauge general consistency.
Reply questions like:
❔ How does the distribution of [numeric variable] examine throughout completely different [categories]?
Superstore Instance:
Our query is: “Do revenue margins differ considerably by area, and which area has the widest unfold?” As a result of this query focuses on evaluating the distribution of a numeric variable throughout teams, a field plot is a perfect alternative. After we take a look at the chart, we see that the North has revenue margins starting from round –$200 to $350, with a median close to $50. Central is extra tightly clustered round its median, and the South reveals a reasonable vary, with dips beneath zero. These variations assist us determine variation, spot potential outliers, and determine the place to research additional.
Field plots are highly effective for evaluating distributions throughout a number of teams. Nonetheless, in case your viewers isn’t accustomed to them, present a short rationalization or go for histograms when exhibiting a single numeric distribution.
Evaluate
Selecting the best visualization at all times begins by clearly defining the query you are making an attempt to reply. Totally different questions naturally result in completely different chart sorts, and clearly matching your visualization to your query is crucial for efficient communication.
With follow, choosing the fitting visualization will turn into second nature. You will really feel assured figuring out you are clearly speaking your knowledge insights, making your visuals straightforward for any viewers to know and act upon.