The fast evolution and enterprise adoption of AI has motivated unhealthy actors to focus on these techniques with better frequency and class. Many safety leaders acknowledge the significance and urgency of AI safety, however don’t but have processes in place to successfully handle and mitigate rising AI dangers with complete protection of all the adversarial AI risk panorama.
Strong Intelligence (now part of Cisco) and the UK AI Safety Institute partnered with the Nationwide Institute of Requirements and Know-how (NIST) to launch the most recent replace to the Adversarial Machine Studying Taxonomy. This transatlantic partnership aimed to fill this want for a complete adversarial AI risk panorama, whereas creating alignment throughout areas in standardizing an strategy to understanding and mitigating adversarial AI.
Survey outcomes from the World Cybersecurity Outlook 2025 printed by the World Financial Discussion board spotlight the hole between AI adoption and preparedness: “Whereas 66% of organizations count on AI to have probably the most vital affect on cybersecurity within the 12 months to come back, solely 37% report having processes in place to evaluate the safety of AI instruments earlier than deployment.”
With a purpose to efficiently mitigate these assaults, it’s crucial that AI and cybersecurity communities are effectively knowledgeable about at this time’s AI safety challenges. To that finish, we’ve co-authored the 2025 replace to NIST’s taxonomy and terminology of adversarial machine studying.
Let’s take a look at what’s new on this newest replace to the publication, stroll by means of the taxonomies of assaults and mitigations at a excessive degree, after which briefly replicate on the aim of taxonomies themselves—what are they for, and why are they so helpful?
What’s new?
The earlier iteration of the NIST Adversarial Machine Studying Taxonomy targeted on predictive AI, fashions designed to make correct predictions primarily based on historic knowledge patterns. Particular person adversarial methods have been grouped into three main attacker targets: availability breakdown, integrity violations, and privateness compromise. It additionally included a preliminary AI attacker approach panorama for generative AI, fashions that generate new content material primarily based on current knowledge. Generative AI adopted all three adversarial approach teams and added misuse violations as an extra class.
Within the newest replace of the taxonomy, we develop on the generative AI adversarial methods and violations part, whereas additionally making certain the predictive AI part stays correct and related to at this time’s adversarial AI panorama. One of many main updates to the most recent model is the addition of an index of methods and violations in the beginning of the doc. Not solely does this make the taxonomy simpler to navigate, nevertheless it permits for a better method to reference methods and violations in exterior references to the taxonomy. This makes the taxonomy a extra sensible useful resource to AI safety practitioners.
Clarifying assaults on Predictive AI fashions
The three attacker targets constant throughout predictive and generative AI sections, are as follows:
- Availability breakdown assaults degrade the efficiency and availability of a mannequin for its customers.
- Integrity violations try and undermine mannequin integrity and generate incorrect outputs.
- Privateness compromises unintended leakage of restricted or proprietary info reminiscent of details about the underlying mannequin and coaching knowledge.
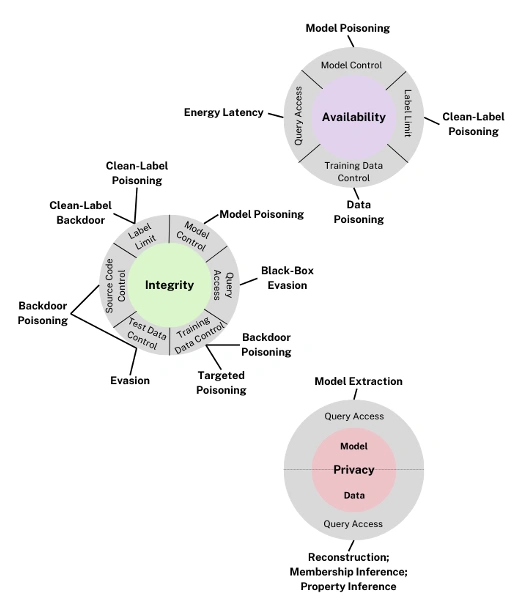
Classifying assaults on Generative AI fashions
The generative AI taxonomy inherits the identical three attacker targets as predictive AI—availability, integrity, and privateness—and encapsulates further particular person methods. There’s a fourth attacker goal distinctive to generative AI: misuse violations. The up to date model of the taxonomy expanded on generative AI adversarial methods to account for probably the most up-to-date panorama of attacker methods.
Misuse violations repurpose the capabilities of generative AI to additional an adversary’s malicious targets by creating dangerous content material that helps cyber-attack initiatives.
Harms related to misuse violations are supposed to provide outputs that would trigger hurt to others. For instance, attackers might use direct prompting assaults to bypass mannequin defenses and produce dangerous or undesirable output.
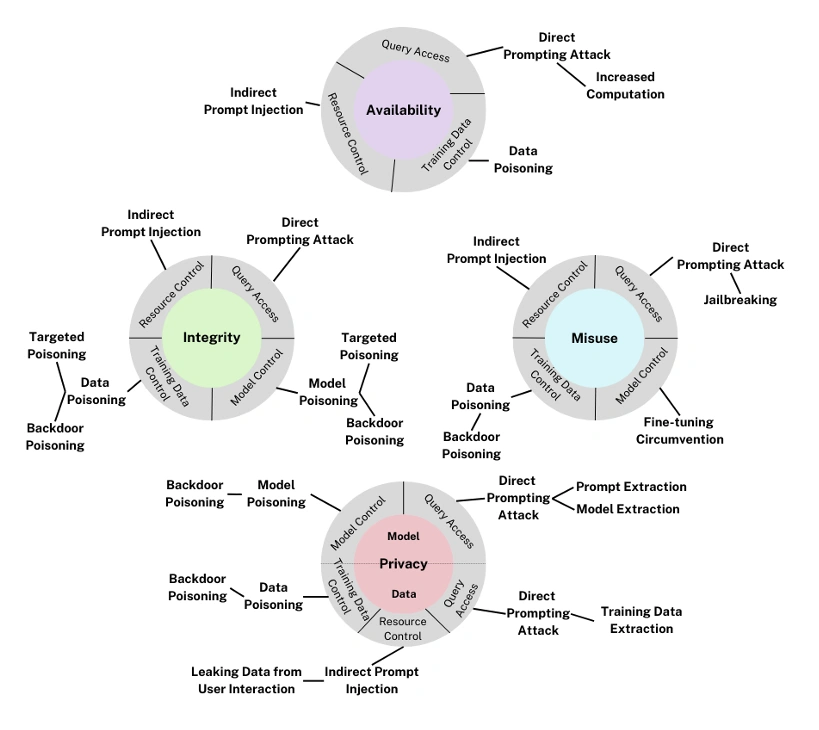
To attain one or a number of of those targets, adversaries can leverage quite a lot of methods. The enlargement of the generative AI part highlights attacker methods distinctive to generative AI, reminiscent of direct immediate injection, knowledge extraction, and oblique immediate injection. As well as, there may be a wholly new arsenal of provide chain assaults. Provide chain assaults will not be a violation particular to a mannequin, and due to this fact will not be included within the above taxonomy diagram.
Provide chain assaults are rooted within the complexity and inherited danger of the AI provide chain. Each part—open-source fashions and third-party knowledge, for instance—can introduce safety points into all the system.
These could be mitigated with provide chain assurance practices reminiscent of vulnerability scanning and validation of datasets.
Direct immediate injection alters the conduct of a mannequin by means of direct enter from an adversary. This may be completed to create deliberately malicious content material or for delicate knowledge extraction.
Mitigation measures embrace coaching for alignment and deploying a real-time immediate injection detection answer for added safety.
Oblique immediate injection differs in that adversarial inputs are delivered by way of a third-party channel. This method will help additional a number of targets: manipulation of data, knowledge extraction, unauthorized disclosure, fraud, malware distribution, and extra.
Proposed mitigations assist decrease danger by means of reinforcement studying from human suggestions, enter filtering, and the usage of an LLM moderator or interpretability-based answer.
What are taxonomies for, in any case?
Co-author and Cisco Director of AI & Safety, Hyrum Anderson, put it finest when he stated that “taxonomies are most clearly essential to arrange our understanding of assault strategies, capabilities, and targets. In addition they have a protracted tail impact in enhancing communication and collaboration in a subject that’s transferring in a short time.”
It’s why Cisco strives to assist within the creation and steady enchancment of shared requirements, collaborating with main organizations like NIST and the UK AI Safety Institute.
These assets give us higher psychological fashions for classifying and discussing new methods and capabilities. Consciousness and training of those vulnerabilities facilitate the event of extra resilient AI techniques and extra knowledgeable requirements and insurance policies.
You possibly can evaluate all the NIST Adversarial Machine Studying Taxonomy and be taught extra with a whole glossary of key terminology within the full paper.
We’d love to listen to what you suppose. Ask a Query, Remark Beneath, and Keep Related with Cisco Safe on social!
Cisco Safety Social Channels
Share: